In recent years, deep learning and neural networks have received a lot of attention. In TED talks, media stories, and industry papers, deep learning has been heralded as the next generation of computing. Indeed, last year’s Turing Award, computer science’s equivalent of the Nobel Prize, went to three researchers who pioneered deep learning.
So, what is deep learning, what differentiates it from other forms of AI, and why is it so hot right now? In this article, we’ll do a deep dive on recent trends and see how companies are leveraging deep learning and neural networks to solve real problems.
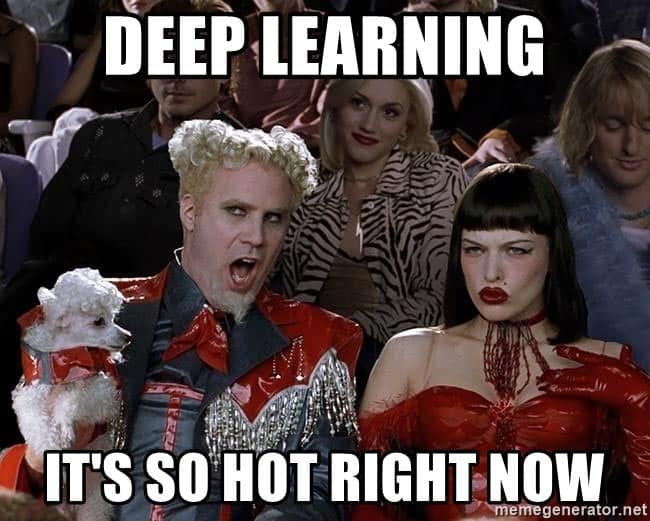
What Is Deep Learning?
Deep learning is a subset of machine learning, under the broader umbrella of artificial intelligence. However, deep learning has some key distinctions from traditional machine learning methods that make it unique in the field.
At its core, machine learning generally is about pattern recognition. Once a computer learns to recognize patterns in a given domain, it can classify new data that it receives based on what it learned from past trials.
For traditional machine learning models, these pattern classifications are usually simple – a single question or problem domain with a specific answer. Humans prepare data, extract features ahead of time, and label it before the algorithm can learn and process information.
In contrast, a deep learning model is an end-to-end problem solver. Using layers of networked nodes, the deep learning model does the feature extraction and identification of multiple patterns all at once. This makes deep learning useful for much more complicated problems. However, because this complex multi-layered network of nodes trains itself over time through reinforcement, it can also be difficult to understand how a deep learning algorithm works behind the scenes.
Emergence of Deep Learning
Since the dawn of the computer age, researchers have theorized about machine intelligence. In the 1940s, the forefathers of modern computing dreamt of having intelligent computers that can learn and intuit solutions to complex problems. Over the 1950s and 60s, the first neural networks emerged, including the Perceptron algorithm for image classification. However, these early cases were very simple and not applicable across wide use cases.
Neural networks reemerged in the 1980s when researchers developed methods for backpropagating parameters in order to build and train multi-level neural networks. This was the era when Yoshua Bengio, Yann LeCun, and Geoffrey Hinton did much of the groundbreaking work in the field. For their work, these three won the 2018 Turing Award “for conceptual and engineering breakthroughs that have made deep neural networks a critical component of computing.”
As the 2000s progressed, techniques matured to allow increasing layers of neural networks. These many-layered networks prompted the move to name this field of AI research “deep learning” since algorithms now processed data several layers deep in order to reach an answer.
In 2012, deep neural networks began to outperform traditional classification algorithms, including machine learning algorithms. This is largely due to increased performance of computer processors (CPUs & GPUs) and larger storage media for retaining huge training datasets. Every year since, deep learning has continued to get better, becoming state of the art for solving problems in many different domains. This explosion in deep learning is largely thanks to improvements in hardware and massive labeled data sets that allow deep learning models to improve quickly over time.
Deep Learning’s Practical Applications
Because deep learning is a problem-solving framework for machines, it has applications in tons of use cases. Choosing a model, creating parameters, and training on a dataset comes with challenges, but the benefits can be enormous when implemented correctly.
Face & Object Recognition
Deep learning neural networks are at the heart of many state of the art computer vision applications. From self-driving vehicles to cashier-less stores, we can use object and facial recognition to automate machine decision making. For example, Tractable is a company that estimates damage after disasters. Their deep learning-powered solution allows them to identify damages and make estimations autonomously in real time.
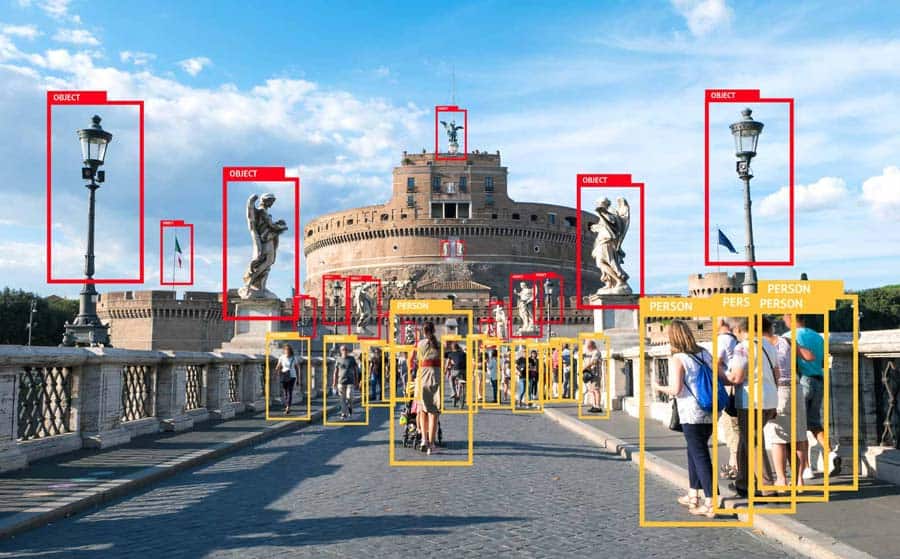
Natural Language & Speech
Another major problem domain for deep learning is natural language processing. Companies like Google and Amazon are on the cutting edge of this research to power the Google Assistant and Amazon Alexa. Additionally, language processing will power innovative solutions for the deaf and hard of hearing. Moreover, we might have AI assistants that can translate between languages for us in real time very soon.
Customer Relationship & Recommendation Systems
This is one area where deep learning is already having a major economic impact. Companies use deep learning to power recommendation engines that lead to higher customer conversions and overall cart values, both online and in-store. For example, we’ve previously written about the growing uses of computer vision in retail. Moreover, neural networks have become increasingly adept at modeling and predicting human behaviour and buying decisions. This allows companies to model and plan for various customer and economic scenarios.
Fraud Detection
We mentioned earlier that deep learning is all about pattern recognition. Fraudulent charges are usually transactions that break those patterns. Companies like Capital One are leading the way in using deep neural networks to detect fraud in real time based on behavioural analysis and statistics about past fraudulent transactions. Deep learning can identify other types of fraud, too. Facebook, Twitter, and YouTube are using neural networks to target fake accounts that are manipulating news and popular opinion.
Bioinformatics & Drug Discovery
Deep learning has many applications in the health field. It’s currently used to do faster, more accurate analysis of medical images like CT scans, MRIs, and x-rays. In addition, neural networks can help find the biochemical targets of new drugs under development, simulating real trails from a computer. Other programs, like DeepMind’s Streams solution, are currently notifying specialist doctors when test results indicate an urgent medical issue. Patients in NHS hospitals are currently being seen more quickly for kidney injuries thanks to DeepMind Streams in a pilot study.
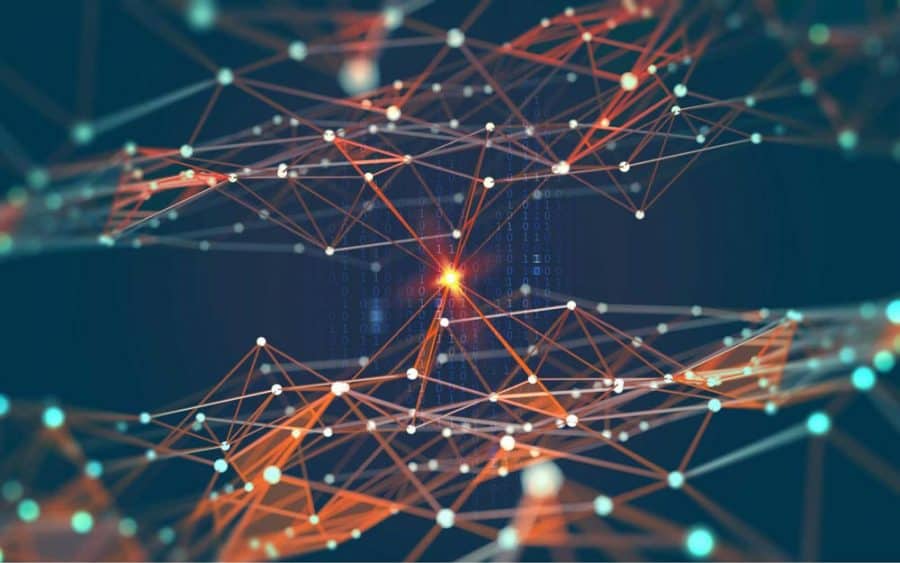
Why Major Companies Are Investing Heavily in Deep Learning
McKinsey estimates trillions of dollars of impacts globally from deep learning over the coming years. Specifically, the research predicts a 1% – 9% increase in revenues for companies that deploy deep learning effectively. However, know-how and infrastructure are key. Deep learning models need to be retrained regularly to stay relevant. They also need tweaking to improve gradually over time and respond to new parameters.
Compared to traditional machine learning methods, however, deep learning has the potential to solve much more complicated problems and intuit new solutions. It requires less upfront work with feature engineering and dataset labelling. However, deep learning also requires large datasets and high-performance hardware in order to work effectively. Deep learning shines in very complex domains where solutions aren’t simple.
What Deep Learning Could Mean for You
It’s still very early in the deep learning trend. While most companies have heard about and are interested in deep learning, few are implementing it yet. There’s still an opening for early adopters to catapult the competition. In a few short years, nearly every company will be leveraging deep learning in some way. The potential applications are so broad.
Deep learning is powerful, but it’s not without its drawbacks. A neural network is only as good as the data it is trained on. If the dataset is biased, so too will be the neural network. It’s critical that companies think about the ethics of deep learning as they’re developing new solutions. As such, a good rule of thumb is deep learning shouldn’t be focused on making more money or extracting value. It should be a public good that makes lives easier for consumers.
It’s tempting to jump into the deep end with deep learning, but that’s a sure path to overwhelm. Above all, your first deep learning solutions should focus on practical benefits for customers in narrow problem domains. If you can effectively leverage deep learning in a specific problem domain that helps your customers, then you’ll be in a good position to make judgments about other, more complex areas in your business that might be candidates for deep learning as well. You’ll also be able to gradually build out your team of AI experts as you scale your solutions.
Keep reading
Computer Vision in Retail: Welcome to the Store of the Future
Get our latest articles and insight straight to your inbox
Hiring Machine Learning Talent?
We engage exceptional humans for companies powered by AI