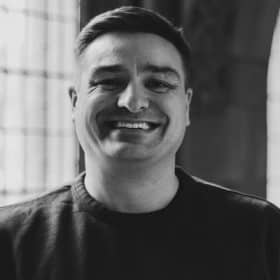
2017 was an important year for artificial intelligence. With increased funding and many major corporations involved in AI development of some type, 2017 was the year that AI became mainstream. It seems every week news articles touted a new application of “deep learning,” “neural networks,” or “machine intelligence.” The fact that these terms have become so commonplace in our lexicon is evidence of AI’s arrival in the public consciousness.
But 2017 wasn’t just a big year for AI in terms of public relations. It was also a massive year in terms of new development. We’ve made significant progress on training networks, curating good datasets, and even creating synthetic data. AI hardware has come into focus for major manufacturers, and we saw an explosion in competition amongst AI chip manufacturers, various sensor and data collection providers, and new startups offering AI consulting or platforms.
Entering 2018, AI is poised for explosive growth. In this article, we’ll take a look at the current state of AI for business and consumers. We’ll also examine the avenues of new development where academics and enterprises will be focusing their energy in 2018. Finally, we’ll zoom out to explore the overall trends in AI and what an AI future might look like.
Real World Business Applications of AI
2017 was the year where it became mainstream for businesses to make investments in artificial intelligence. Many of these businesses have found use cases for automation in marketing, sales, and advertising. Other companies have used AI to investigate bottlenecks and inefficiencies in manufacturing, shipping, or agriculture. Still, others use AI to complement their employees’ capabilities, making tasks like research, compliance, and even creative work less demanding for employees so they can focus on the important tasks that computers can’t do.
There are many business applications of AI, but here are some of the most interesting emerging ones.
Personal Digital Assistants
For knowledge workers, so much of the day is consumed with administrative and research-based tasks. Finding documents, setting up appointments, and sending emails are all critical components of modern office work. However, experts in artificial intelligence are questioning whether some of these tasks might be able to be automated. An AI personal assistant could find information quicker than you probably could. It could also pre-draft emails for you, suggest meeting times that work for all parties, automate your record keeping and time tracking, and take care of reporting and compliance concerns.
Journalism
At a time when trust in the press is at an all-time low, it seems like there’s a role for independent verification of new stories and sources. AI could provide an answer. A team at Reuters is implementing AI algorithms that track social media for breaking stories and try to verify their legitimacy. This allows the Reuters team to discover, verify, and share stories more quickly. AI could also lead to a new brand of personalised journalism that takes into account stories that affect your family, community, or personal interests. Of course, this type of curation runs into ethical questions about bias and guaranteeing outside viewpoints. However, there’s an argument to be made that a well developed news algorithm could provide those outside opinions and marginalised voices with better consistency.
Law, Accounting, & Business
It used to be that lawyers focused on the risks of incorporating artificial intelligence into your business practices. Increasingly, legal experts have begun to question whether it might be riskier to not use AI within the business. AI has many benefits for companies, not least of which is automating and standardizing regulatory compliance. Allowing an AI to monitor your business could mean discovering anomalies and errors more quickly. However, using AI still comes with concerns about what the AI knows, how it accesses that information, and how secure it is.
Production & Supply Chain Management
The last few years have shown the big data movement coming into full form. One side effect of companies collecting so much data is a lot of it goes unanalysed. There are only so many data scientists capable of parsing the information. Machine learning can help with this, drawing insights from data over the course of thousands of model tests and observations. The result is companies are seeing greater productivity on the production line and more streamlined supply chain logistics.
Consumer Perceptions of AI are Turning Positive
Just a few short years ago, talking about artificial intelligence with the average consumer conjured up ideas of sentient robots taking over society. While the debate has now changed to the ethical questions of artificial intelligence. Now, recognition of AI is commonplace. Artificial intelligence is mainstream. As such, consumers have become increasingly comfortable with smart devices in their home, car, and pocket. Websites regularly give their customers smart recommendations based on past behaviour. Language and image processing allow Alexa to learn what you like, Google to predict what you’ll want to say in your reply email, and Facebook to tag you in photos you didn’t previously know about.
These everyday applications of AI make life simpler and more efficient. In so doing, they inspire consumer confidence in and familiarity with AI. As we become more reliant on AI to help us get through life, its effects on us become more profound. AI designers will need to pay attention to make sure AI reduces bias and fosters empathy, instead of exacerbating prejudice in an echo chamber. It’s important to remember that AI is only as good as the data we give it. AIs have been shown to hold the same biases as the society creating it. Creating neutral datasets and algorithms is one of the key ongoing challenges facing AI in 2018 and the foreseeable future.
What to Expect in 2018
Computer scientists, developers, and theorists are hard at work improving machine learning systems to be more reliable, flexible, and responsive to our needs. In this article, we’ve already touched on a few key challenges facing AI. Here are a few more challenges that I expect researchers to run up against, and make progress on, in 2018.
Deep Learning Theory
We’ve been creating deep neural networks for a while now. They’re essentially complex networks that mimic the structure of the human brain. Neural networks can learn information from image, audio, and text. However, we still don’t really understand how they do it. New theories being tested and expanded in 2018 show promise for explaining this phenomenon. If we can understand the black box of neural networks, it could yield insights into the best ways to build and operate future networks.
Synthetic Data & Model Transfers
Machine learning relies on having lots of accurately labelled data in order to train algorithms. While we have an abundance of data, most of it is not well labelled. Labelling usually requires humans to analyse the data first, decide on the correct answer and then use the set of data and correct answers to teach the algorithm. Having humans label thousands of data points is time-consuming. Recently, researchers have begun to create synthetic data for training machine learning algorithms. It’s data that follows the same patterns as real data, but it’s properly labelled from the outset. We can produce synthetic data much quicker, leading to faster model training.
Another avenue for faster model training is taking an algorithm that works well in one domain and transferring its capabilities to another. If we can get better at model transfer, then there’s no need to write and train a new algorithm every time we have a new application of machine learning. By creating data and using pre-trained models we reduce the burden of real data required. This allows machine learning to extend to realms previously lacking in historical data.
New & Better Hardware
Moore’s Law will continue and along with it processors, servers, software frameworks, and AI-specific hardware will continue to improve. This will speed up algorithm training and experimentation. More accurate and cheaper sensors will also likely come out, making data collection cheaper and/or higher resolution.
Better Developer Tools
AI development is expanding and will soon become a mainstream skill for software engineers. Expect deep learning libraries like TensorFlow, Caffe, PyTorch, and BigDL to continue to grow in users and usefulness. Other tools will likely come in the near future that simplify model training, architecture, and deployment, expanding access to machine learning outside data engineers and data scientists.
The Big Picture: Imagining an AI Future
AI has a role to play in almost every major industry. Anywhere where we gather data in order to make decisions, AI has a use case. As the cost of developing and deploying AI continues to decrease, it will become increasingly practical to use it even in small applications. The overall effect is likely to be transformative on society, the economy, and our day-to-day lives.
John Scully, the former CEO of both Apple and Pepsi, had this to say after seeing the AI applications on exhibit at this year’s CES conference:
“AI is going to be foundational in every industry. I’m seeing it in fintech, market tech, health tech…. It’s one of those fundamental changes. In the previous industrial age, it was all about electricity and oil; in the future [AI is] going to be a commodity that will be deployed in many, many different ways, and will be something you can just plug into.”
It’s an exciting time to be working in AI. The energy around the technology is palpable, and investment is pouring in. Over the next few years, consumers will begin to expect that companies are implementing AI. The best technologies will take artificial intelligence as an essential resource for their platforms. With that expansion comes a growing demand for expertise in AI, making this a great time to transition early to the field.
Get our latest articles and insight straight to your inbox
Hiring data professionals?
We engage exceptional humans for companies looking to unlock the potential of their data