Deep learning, a subset of artificial intelligence that uses unsupervised learning to process data within a hierarchical structure (similar to the way a child learns), is perhaps the most powerful forms of machine learning technology. The ability of a deep learning system to continuously improve its knowledge in close to real time, independent of manual programmer input, makes it perfectly suited for use ‘in the wild’, without the need for ongoing professional intervention. As such, deep learning is the perfect partner for improving the increasingly pervasive technologies of augmented and virtual reality.
Deep learning relies on an influx of large volumes of data in order to expand its knowledge and strength. With virtual and augmented reality, a steady stream of unstructured data (principally, but not limited, to image and video) is pumped into the system for it to work through, with the algorithm translating the data into practical instructions for how the VR/AR system should run.
The capabilities of deep learning systems are continuing to evolve. Speech and image recognition, in particular, are increasing in sophistication at a rapid rate. Financial, storage and processing costs are decreasing, and richer data streams are becoming available as a result of expanding network bandwidth. These developments not only catalyse the uptake of deep learning in enterprise and industrial use alone, more powerful deep learning systems have a knock-on effect on assisting with the expansion of use for VR and AR.
Indeed, in order to produce any form of interactive virtual or augmented reality some degree of artificial intelligence is necessary. If we consider AI at its most basic definition, an algorithm that is able to compute using its own knowledge base can be considered artificially intelligent. Whilst 360-degree imagery and video that can be accessed via a headset requires no AI to function, both VR and AR gaming – for example – does. If you consider movement and interaction with the virtual environment, or with real-world objects in AR, there is incoming data from the outside world that needs to be processed and reacted to within the app.
Hand-and-Eye Tracking in VR with Deep Learning
Systems that allow people to interact with virtual environments will require computers that are able to interpret human reactions, such as hand and eye movement.
Regarding hand movements, there are millions of poses and motions that the human hand can make. All of these motions and poses need to be taken into account when designing a spatial user interface where the user is interacting with the computer in space (rather than through manual clicking, keyboards, or touch screens).
For this, it is entirely necessary to have a sophisticated deep learning system that will quickly learn and understand each of these endless poses and motions and how they apply in the virtual world. To do this, a high-end VR system will work with deep learning algorithms in the form of convolutional neural networks, a type of neural network that is particularly adept at recognising spatial relationships.
Eye tracking is another deep learning-powered functionality that is fast being adopted as a logical necessity for evolving VR and AR systems. At CES2018, a growing start-up in the area of VR eye tracking, Tobii, made a substantial impact on those who tried out its hardware at the event. Journalists reported enthusiastically on the positive difference the Tobii eye tracking system built to their experience of VR. What made their experience so compelling?
The principal benefit of eye tracking in VR is in what is known as foveated rendering. With foveated rendering, only that which is in the user’s direct gaze is rendered in full resolution (in the same way that your eyes see in real life). As system resources are only focused on one area of the visual field, there is a significantly less drain on bandwidth and processing. Incredible visuals can thus be created without the risk of slowing down the experience, resulting in more realistic and intuitive user experience.
Beyond foveated rendering, however, there are other aspects of eye tracking in VR and AR that vastly improve both the user experience and the opportunities for the capturing of data gleaned from the user. And these aspects go far beyond the applications in gaming (which are, incidentally, many).
Eye-Tracking in Retail Market Research
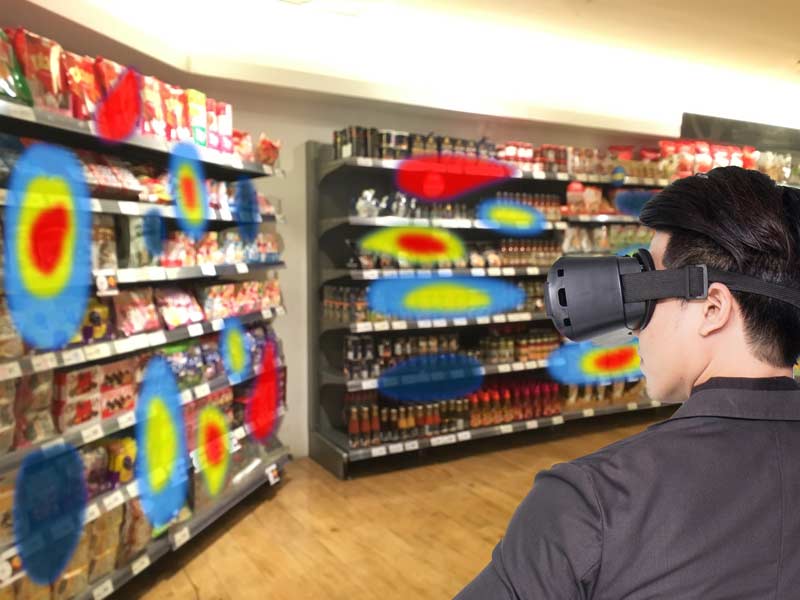
Virtual reality is proving itself a particularly useful technology for retailers (and marketers in general) in market testing environments. Retailers currently spend a lot on market research, which can involve lots of time and resources. For example, when testing new layouts for bricks-and-mortar stores, in the past it was necessary to build physical prototype spaces. Because of the cost and time involved, it was thus only possible to test perhaps one or two spaces in prototype mode to gauge consumer sentiment. Building virtual prototypes, on the other hand, is significantly less cost- and resource-intensive, meaning that a higher number of prototypes can be market tested. This is where eye tracking comes in.
Rather than conducting interviews and getting verbal feedback on prototype retail spaces, eye tracking combined with deep learning systems allows market researchers to discover much more in-depth information about how users respond to areas. By analysing data generated on where user gaze travels within a simulation, the researcher can ascertain the best location for planograms, point-of-purchase displays, and to follow the most popular user journey throughout the store and know what products perform best in which spaces.
More than gaze direction tracking, eye tracking sensor hardware within a VR headset can deliver data on other physiological responses acquired through monitoring responses such as pupil dilation (which is a proven indicator of emotional engagement, as well as mental strain).
Deep Learning In VR/AR Training Simulations
These deep learning systems that work with hand and eye tracking are not just useful for retailers and marketers, however. The applications in education and training situations are immense and do not only apply in immersive VR, but also in AR (where virtual elements are overlaid onto real-world vision).
When gathering data from virtual training simulations (e.g. firefighting, military, emergency responders) can aid in the training process. Understanding how and why trainees are failing at specific tasks or providing extra information where necessary to boost the power of the training, without the need for human trainers to intervene, is a resource-efficient, time-saving use of the technology.
Deep Learning in Augmented Reality
Augmented reality glasses are on their way in, and already proving useful in jobs such as asset maintenance, construction, emergency response, military and surgery. By overlaying vital information gleaned from image and speech recognition data (processed by connected deep learning systems) onto the worker’s field of view, tasks can be completed with increased accuracy.
Equally, hazard warnings can be issued where necessary. Soldiers with augmented reality displays (either built into their helmet, glasses or goggles or in contact lenses) powered by AI can be helped to choose the best course of action. A deep learning system can run millions of simulations, compare the current situation being faced by the soldier to previous situations in its archive, and provide deeply and accurately assessed information as to how the soldier should act. Such as system could also highlight potential present dangers before they are perceived by the user – great for soldiers, but also to protect cyclists from other road users (see Garmin’s radar-equipped Varia Vision as an example).
Deep Learning, AR, and the Consumer
Whilst virtual reality has its own swathe of uses, augmented reality – whether smartphone or wearable – is set to have a powerful impact on work, leisure, and society in general. Tim Cook has even gone so far as to say that “augmented reality is going to change the way we use technology forever”. And unlike VR, whose need for deep learning integration is unnecessary for some of its functions, augmented reality cannot function without some form of integration with artificial intelligence.
Consumer-facing retail applications for augmented reality are already being realised. These applications will only grow as adoption of AR (probably with wearables) expands, but even on the smartphone, retailers are rapidly picking up on the advantages AR offers.
Augmented reality has powerful implications for omnichannel marketing, allowing retailers to expand their customer service and personalisation (while gathering valuable data on customer preferences). With a branded smartphone app, users can access exclusive, personalised discounts and offers in-store by holding up their phone within the physical environment.
Items that shoppers show interest in can be monitored and recorded, and additional information on those items (with alternative versions of the product or complementary items to consider) can be displayed in AR. We are also beginning to see the emergence of augmented reality fitting rooms (both installed in-store and accessible on mobile).
Let’s also not forget the well-documented uses of augmented reality in the furniture retail sector, where customers can view furniture products in their own homes with the use of their smartphone camera.
All of these applications rely on deep learning systems to work with data gathered to improve user experience and feed information back to brands to strengthen product offering and thus sales and ROI.
Deep Learning, Augmented Reality and Business Use
The applications we have looked at for augmented reality in retail and training can also apply to business use cases. Face recognition AI (with data provided by AR systems) can help us to identify people to speak to at conferences, as the algorithm produces and displays information on other attendees (such as their name, job role, etc. – depending on whether they have enabled access to this information, of course) on either your smartphone screen or HMD (head-mounted display). Telepresence at video conferences can be enhanced in the same way, as well as accessibility for deaf or foreign language-speaking attendees, as translation and subtitling capabilities grow.
Rather than seeing AR/VR and deep learning (or AI/ML) as separate technologies, we should be considering them as symbiotic. Without artificially intelligent systems in place, both virtual and augmented reality would remain incredibly basic. Deep learning is playing a fundamental role in facilitating the development and adoption of virtual and augmented reality technologies and will continue to do so with increasing levels of functionality, accuracy, and power.
If you would like to discuss anything from this article, please feel free to reach out to me.
If you enjoyed this article, you may be interested in signing up to our monthly newsletter. Please sign up here.
Get our latest articles and insight straight to your inbox
Hiring Machine Learning Talent?
We engage exceptional humans for companies powered by AI